article
Bridging the conversational gap between humans and AI with natural language understanding
Women in Tech series
May 03, 2022 • 8 minutes
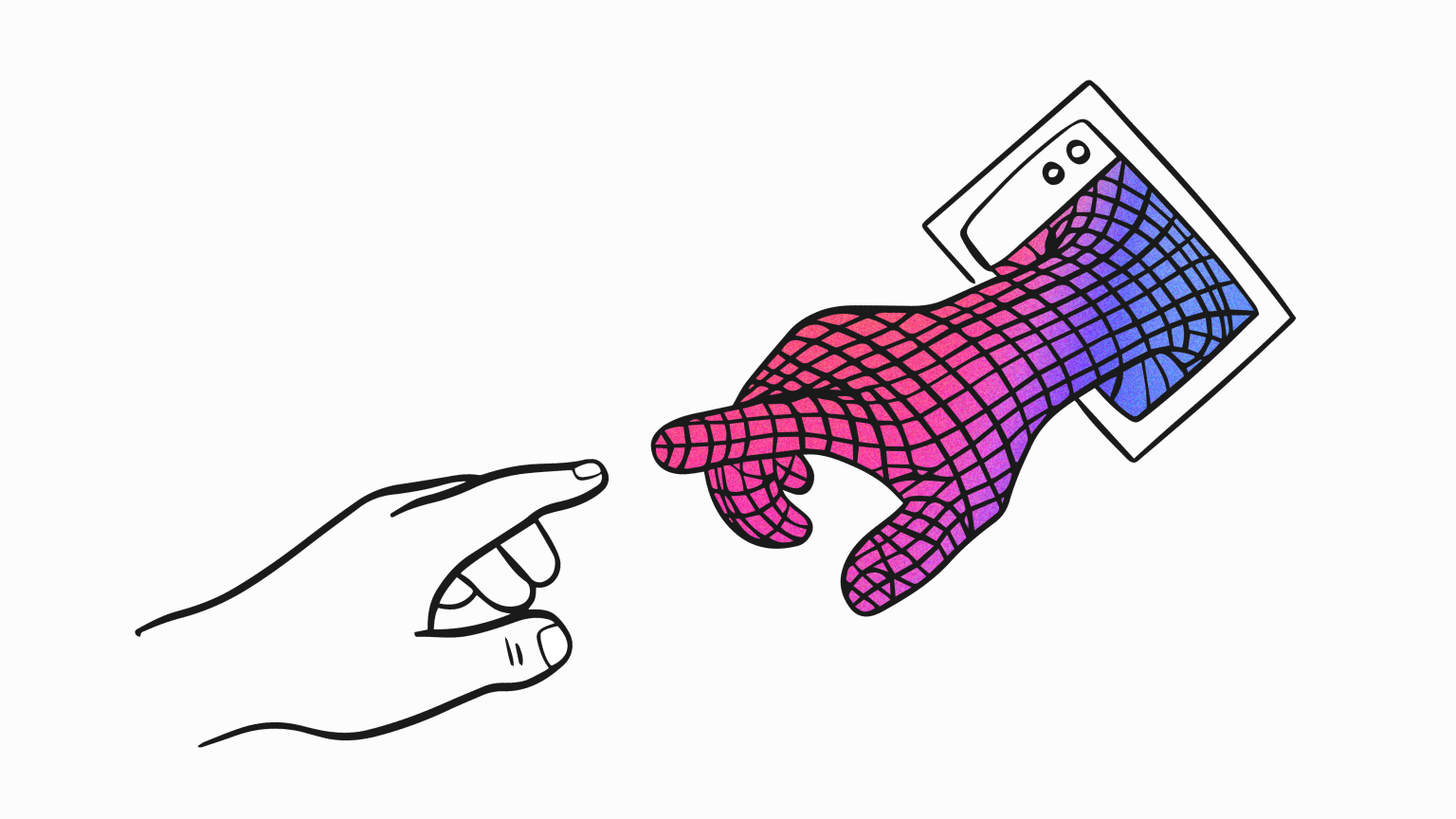
Computers don’t understand language. That’s where we, Anna and Dalia of the Taxonomy team, part of the LivePerson Data Science team, come in. We work in a field specifically called Natural Language Understanding (NLU). In our roles, we straddle the two worlds in an effort to help computers understand human conversation, bridging the gap between the artificial intelligent systems and the natural language of humans.
We sit between humans and artificial intelligence, translating what people are thinking and asking when they interact with Conversational AI throughout their daily lives. Our goal? To increase virtual assistants’ comprehension and ability to answer questions in ways that match the consumer’s intent. After all, as humans, we can go beyond sentiment analysis to articulate why answers are good or not very good. We spend a lot of time reading customer messages with brands, keeping an eye toward grouping and categorizing that unstructured data in ways machines can understand…all to help make the brand-to-consumer “auto-magic” happen.
Read on for a brief synopsis of how we got here, and learn about the work we do today to create quality Conversational AI messaging for brands and consumers around the world.
Meet Anna Folinsky
I joined LivePerson at the beginning of 2022, bringing almost 20 years of experience in labeling data for use in machine-learning models, beginning with geographic information and search. More recently, I spent several years learning all aspects of Conversational AI. My background is in cognitive science and chemistry, allowing me to combine the core aspects of human cognition and practical experimental design into my work of making sense of human language and interactions.
Meet Dalia Levine
I joined LivePerson in 2021 with over 15 years of taxonomy experience in various companies and fields, most recently from HBO/WarnerMedia. My experience is in classifying information either in a conversation or across multiple sources, documents, and any type of media. I contributed to the implementation and creation of international metadata standards, and I contributed to the use of metadata standards to capture knowledge and streamline workflows. I trained as a librarian, and I geek-out with fellow information architects about ways data is categorized. I often say:
“When I organize information, I help to get the computer to understand what you really mean.”
What we do to improve LivePerson’s natural language understanding
As a company, LivePerson cares about applying Conversational AI respectfully. One way to think about our work is as a united front with the efforts of EqualAI® to implement better ways for systems to work with humans. With our varied experiences in search and classification of documents and machine learning — and structuring and processing data based on customer intent — we understand the “gotcha” moments that can happen when categorizing and classifying data and how to look for it in technical processes. We understand how language shifts and changes over time. We apply the contexts in which interactions and information are processed by people.
In other words, we consider specific details about what people “really mean” and define frameworks to capture that meaning for natural language understanding. These frameworks allow us to surface and distill our intuitive understanding of layers of human meaning. The process creates usable outputs that language models can interpret and respond to.
For example, the exact same set of words can mean different things in different contexts. This is generally understood, but part of natural language understanding and natural language processing is thinking explicitly about what happens during such interactions. Someone saying that they are cold has a surface meaning — that person is feeling cold — but you may respond differently depending on the situation. If you are in your living room, you might ask the person who is cold if they want you to turn up the heat. If you are out for a walk, perhaps you would suggest walking faster or maybe just commiserate. In neither case did the speaker ask you to do anything. Both content and context matter in conveying meaning.
Likewise, if someone comes into a conversation with their internet provider and says that their internet is slow, they have only given us a “surface” factual statement and have not asked us to do anything in particular. However, almost any person reading that statement would interpret this (a) as a problem and (b) as a request for the internet provider to fix that problem. That same person uttering that same statement to their bank, however, may simply be apologizing for why they are being slow to respond — or they may even be in the wrong place! There is so much information that we continuously draw upon to infer the actual goal of any given human written or spoken word.
We cannot possibly capture every single piece of that information for a computer to digest. We instead distill the key pieces of content analysis into what is most valuable and actionable within a given system. For instance, we consider the goals of the specific product we are working with. Different systems might need to route customers, judge customer happiness, answer customer questions, or paraphrase customer conversations.
The value we bring to natural language understanding
At our core, we bring curiosity and the drive to understand things. We share a desire to solve problems and “figure it out” with our teammates. Our unique addition is merging the language and human side into the greater technical, natural language processing ecosystem. In our roles, we combine a deep interest in the underlying meaning(s) of language use with the ability to describe and define what those meanings are. This skill lives right at the border between qualitative and quantitative reasoning.
We often do not stop and think about how we organize things, even though that is a key part of our expertise. Therefore, when we try to get others to understand what we’re doing when we look at a chunk of unstructured data or information taken from a conversation, we spend time thinking about what someone is “really trying to say.”
Every time someone communicates with another, that person has a purpose. When Anna was working in search for maps, for instance, she had to start from the thinking that when someone enters in a specific search, they know what they want. Her job was to try to interpret or figure out what that “want” was. Of course, one person may mean something else than another person using the same words.
One of our go-to-examples is searching for Italian food. One person may be perfectly happy with a pizza joint as a result. Another person, however, would say, “No, no, I meant a sit-down restaurant with forks and knives — I want pasta.” What each person wants, that is their “intention” — and we need to have some way to identify and respond appropriately to that. We also might think that while “Italian food” is open to the concept of pizza, someone searching for an “Italian restaurant” is probably less likely to mean that. There are a lot of subtle connotations in what people say, even when they are saying something similar on the surface.
Peeling back the layers of meaning in human language
There are always layers of meaning to what people want and how they expect it to be returned to them. People have an intuitive understanding of these multiple layers of meaning. When we are developing virtual assistants and machine-learning chatbots to interact with humans, we want the artificial intelligence to be able to act as a substitute for a human partner. So, the computer will hopefully act like a competent librarian, a helpful friend, or a useful confidant. To do that, these computer systems need to be able at some level to parse and understand the “real meaning” of what a human is saying — but computers cannot naturally do that.
One of the core needs of our job with natural language understanding is to think about those sublayers of meaning and bring the subtext into text. It’s more than entity recognition, speech recognition, language translation, and processing syntax and semantics. We need to surface the underlying meaning of the request, the user’s intention, and process it into some type of structured data so that the computers have a fighting chance to be responsive in a way that we need them to be.
Part of the reason this is hard is that most of the things we ask computers to do are things that humans are not good at, either!
Doing really massive numbers of computations, sorting lots of data, doing repetitive work, doing lots of technical things like that, that’s what computers do. But understanding language…this is something that humans are good at, although imperfectly. We’ve all misunderstood people or made grievous errors in judgment. Think about it: The entire sitcom genre is predicated on silly misunderstandings. But with NLU technology, we’re asking computers to do something that doesn’t align cleanly with the way that computer science architectures process information.
We need to take the subtext-into-text output and put it into some structured data format that machine-learning systems can use to teach chatbots to be more responsive, more useful partners to humans. After all, when computer software doesn’t act in a way that we expect, we don’t want to work with it. We don’t have a natural way of interacting with it. So in our natural language understanding role, we really serve as this translation layer: thinking about, finding, and labeling these core concepts of what a person is trying to communicate. We are surfacing the gems and rinsing away the dross to get to the parts that are really necessary.
Computers don’t hear subtext. Computers don’t hear sarcasm. Computers don’t hear jokes. Computers see only what is in front of them, and that’s not enough for how most neurotypical humans communicate. We are asking computers to do something hard, and it’s our job to help them do that.
Our final thoughts
Since computers don’t understand human language, are unable to read between the lines, and don’t understand sarcasm or subtext, we fill those voids with natural language understanding. For a computer to interact with us through all of our complicated, messy, emotional, multi-layered communication, someone needs to sort through all the meanings and distill it into something a machine-learning model can use.
We focus on what people “really mean” and define label frameworks to capture those meanings. These labels distill the key pieces of information that are being communicated and allow us to translate all the different kinds of data that people communicate into outputs that computer models can interpret and respond to.
Through our work at LivePerson, we have a shared goal: Make AI-powered conversations smarter, more empathetic, more real, in a simple effort to benefit the user and make them feel seen and heard.