article
How generative AI tools impact the consumer experience and benefit CX leaders
Explore how to use large language models and other AI tools for business
April 17, 2023 • 9 minutes
April 17, 2023 • 9 minutes
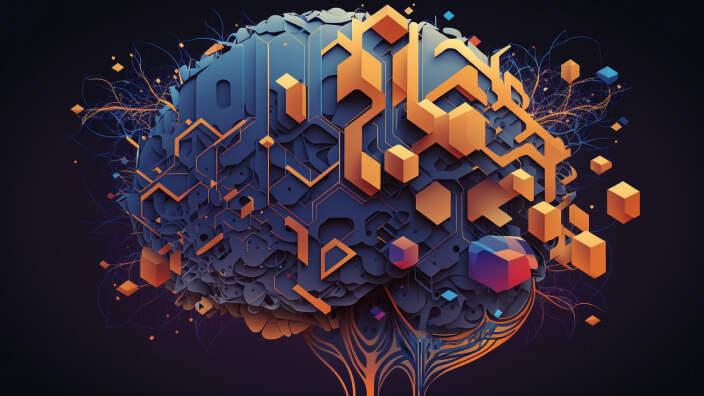
From the rise of human-like chat interfaces like ChatGPT to popular photo editing apps like Lensa AI, generative artificial intelligence (generative AI) has quickly become the next significant transformative technology shift since the iPhone. It’s impossible to browse without hearing about the trending subject in mainstream media. But where does the context of generative AI tools shift from applications that write poetry and edit photos to practical, impactful benefits that transform brand and consumer experiences?
In this blog post, we’ll cover the potential of generative AI in practical use cases for customer experience and benefits it can bring to brands’ bottom lines to drive better business outcomes.
The dream of automated human-like conversations
Businesses have always dreamed of creating automated human-like conversational experiences for all their customers so they can interact at scale. But in the past, it has been expensive and nearly impossible to do so. It is challenging to manually design and program dialogue systems by hand to comprehensively understand and address all user queries. This was limited by machine learning models and their natural language processing (NLP) techniques: Over the past two decades, techniques like neural language models and generic neural networks for NLP could not consistently generate human-like conversations, falling short of fully capturing the complexity of business and consumer conversations. However, this dream has been made possible with the introduction of the Transformer architecture in 2017 and recent developments in large language models.
Large language models: An inflection point
Large language models (LLMs) are a type of generative AI model that focuses on generating and understanding text, trained on billions of words to identify understand the underlying patterns. With these recent developments, we have reached a reality — an inflection point — in the evolution of artificial intelligence, where AI can understand consumers, provide natural and personalized responses (leveraging brands’ existing knowledge sources), and launch in a matter of days, not months. This inflection point is where brands must make the decision to move ahead or be left behind. Brands that choose to move ahead will be at a significant advantage over their competitors.
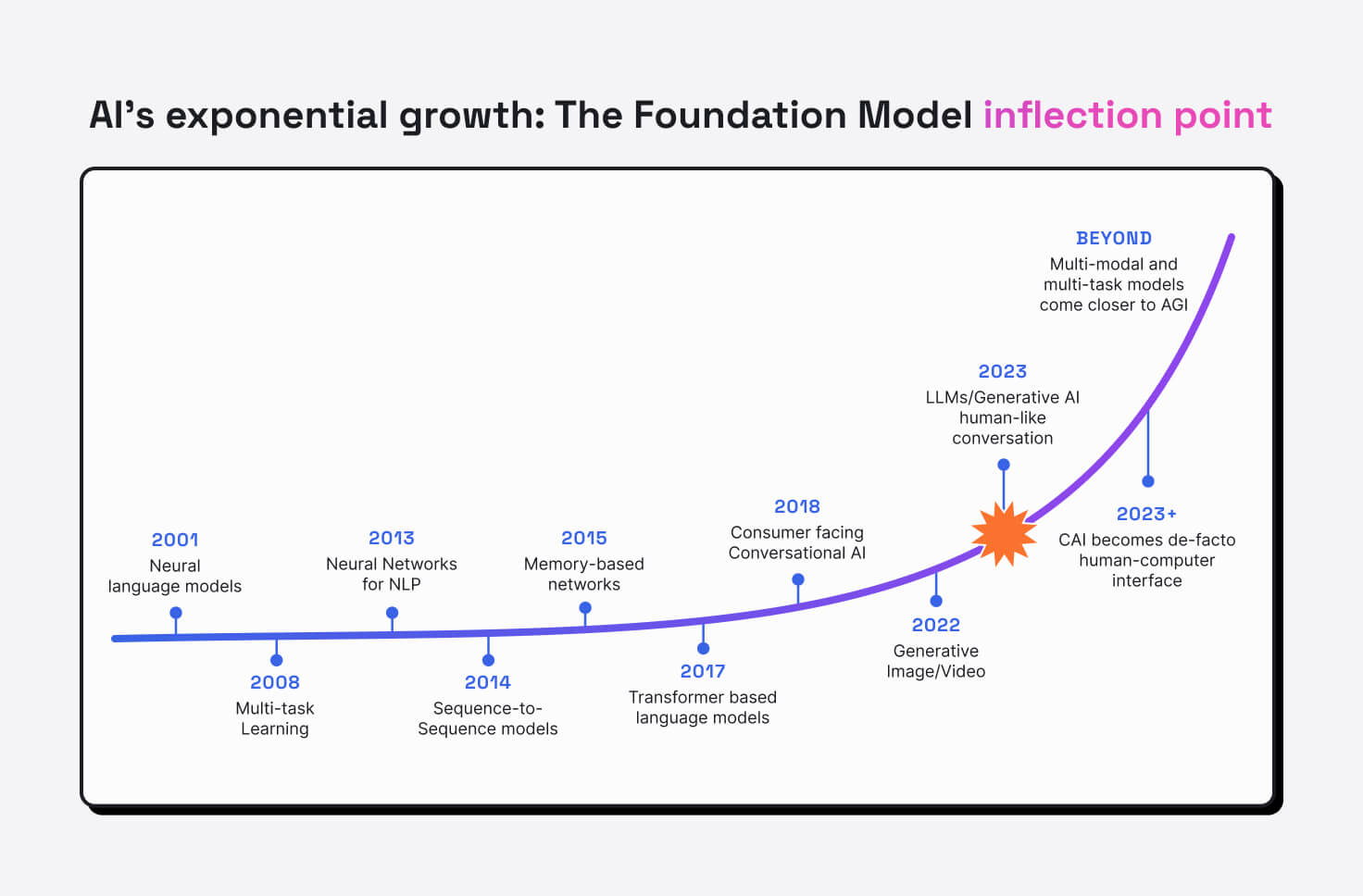
Generative AI challenges for enterprise customer engagement
While generative AI models like LLMs have proven impressive in generating human-like conversations, the challenge we now face is that they are not fit for enterprise customer engagement out of the box. There are four primary issues that must be addressed:
1 – Bias
LLMs can include bias, whether through toxicity, hurtful language, or polarizing responses leading to unintended consequences. We see this especially in the context of decision-making applications, such as hiring or lending, which can result in legal and reputational risks for enterprises. Search “bias in generative AI” and you will be provided with an ocean of examples of AI making biased decisions based on the training data inputs provided to create the model. Our natural bias influences our decisions around what data to include in the generative AI model, more often than not without our knowledge, resulting in real-world impacts to consumers and brands.
Explore LivePerson’s Responsible AI page for more details.
2 – Inaccuracy
Using LLMs for business content creation with little or no customization or fine-tuning will result in responses that are too generic or even irrelevant to your products or the consumer needs. The LLM might generate product descriptions that are too technical or too vague, or that do not accurately convey the unique features or benefits of your products. While LLMs offer a lot of potential for generating natural language text, they are not inherently tailored to specific use cases or industries.
In fact, sometimes large language models can “hallucinate.” Hallucinations, also known as “textual hallucinations” or “generated text errors,” are a phenomenon that happens when a language model generates text that is inaccurate or not relevant to the context or task at hand. Often the response is amazingly confident, even though it is completely wrong. Companies need to customize and fine-tune a large language model to their specific needs in order to achieve optimal results.
3 – Limited actions
LLMs do not have the built-in ability to understand and interact with the specific business processes and enterprise-grade systems that a company uses to manage operations, which are required in order to use LLMs effectively in a company’s workflow. If you wanted to use an LLM to generate automated responses to customer complaints, the LLM would not inherently understand how to integrate with your complaint management system and follow the appropriate workflow. The LLM would simply generate a response based on the input it received, without any understanding of how that response fits into the larger context of the company’s workflow.
4 – Isolation
Large language models, such as GPT-3 or BERT, are designed to analyze and generate human-like language. However, they are not typically connected to inventory systems and do not have the capability to perform orders, track orders, or check if something is available for customer service. For example, let’s say a customer contacts a company’s customer service chatbot and asks if a particular item is available in a specific size. An LLM powers the chatbot and can understand the customer’s question and respond with well-constructed natural language; however, the LLM is not connected to the company’s inventory system, so it cannot check the availability of the item in real-time, making it impossible to take action on the customer’s behalf.
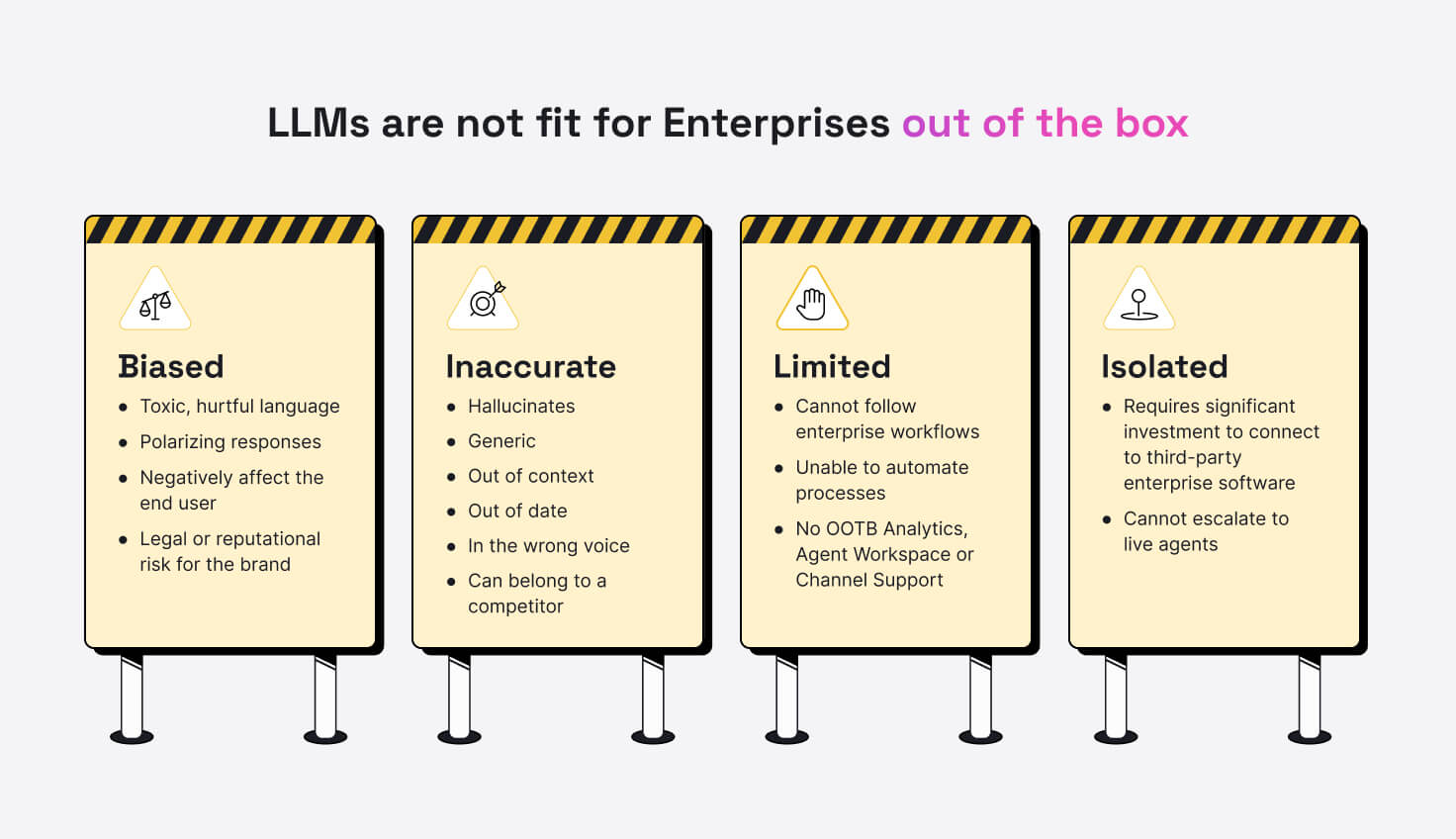
How to help mitigate risks and improve consumer experiences
So, with all of these challenges, how does a brand mitigate the risk, and ensure they are providing amazing, accurate, unbiased responses to consumers? There are several generative AI tools available today to help with this challenge, and here are three ways to start:
1 – Improve training data
First, brands need to control the data being used to train the model. Large datasets can be tricky to work with, but they must be refined to only include accurate, unbiased content, which is used as a source for the LLM to learn from. These datasets should consist of carefully curated content verified by your legal, brand, and customer experience teams for accuracy with enough detail to craft relevant answers for your customers.
It is not as simple as grabbing your existing set of FAQs. You need to make sure the content is updated, current, and accurately reflects your brand. This extends to alternative datasets, such as conversation transcripts from voice or messaging programs. Your best agents can train the AI just by nature of great conversations. Selecting the best samples from these agents to help to shape the generative AI models will result in better responses.
2 – Verify AI-generated content generation
Next, controls have to be put in place to verify the AI-generated responses before sending them to the consumer. As generative AI technology evolves, we will see more of these tools automated out of the box, but until then, there is no better verification than having a human in the loop.
LLM-generated responses should initially be threaded through a human agent before they connect to a consumer. Agent assistance features allow human agents to verify, correct, or reject the response before it is sent to the consumer. AI-powered asynchronous messaging programs provide an excellent structure to put humans in between the LLM and your consumers. Once you are ready to expose your LLM to external customers, you need the ability to escalate quickly and gracefully to a human if the AI provides incorrect answers or goes off the rails, and your human agent needs the ability to report the point of failure for your team to address with the AI. Humans in the loop are a critical first step to protecting your consumer experience and brand reputation as you shift into the world of generative AI.
3 – Start with internal generative AI tools
Finally, as you map out your approach to leveraging LLMs, consider internal, low-risk engagement programs that help you learn while limiting the external exposure to your brand. Internal messaging programs like human resources, IT ticketing, and internal support are great ways to create models in a controlled environment where your existing employees can get the answers they need and provide feedback on the performance of the AI tool. As you get comfortable with creating these models, you can extend the capability to the agent assist tools referenced earlier and, ultimately, to your consumers.
The potential benefits of using LLMs and generative AI tools
If you use a good foundational dataset and put in place controls to protect the experience, the potential benefits of using large language models and generative AI are limitless. Consider just a few of the powerful benefits you can realize:
- Time to market: With generative AI, the paradigm of bot building changes significantly. Gone are the days of hand-crafting complex dialog flows and conversation design from scratch. Using LLMs to answer the simple questions takes hours instead of weeks, getting you to results faster than ever.
- Contact center efficiency: Large language models have the potential to provide higher automated containment rates than traditional dialog based bots. Many of our consumer-to-brand conversations today can be solved with self-help tools, but we struggle to contain those conversations with automation because it is difficult to understand the consumer. Complex NLU models still struggle to understand, but generative AI models perform significantly better at not only understanding the consumer’s natural language, but finding the right content and reshaping the text into a structure that is easy to understand and act upon, taking containment to a new level.
- Personalized, next-level consumer experiences: The ability of LLMs to craft natural-sounding responses makes it very easy for a consumer to interact with the AI. It feels natural, comfortable, and human-like. The AI responses are applicable to the tone of the consumer and can be light-hearted or empathetic in a way appropriate to the consumer problem. They even have natural small talk, making it easy to interact in different ways. Plus, they retain context, so people don’t have to repeat themselves and can continue a thread of dialog that feels very human. This results in consumer experiences that are way beyond the scripted dialog flows we see in traditional bot-building — we’re talking next-level experiences.
Generative AI tools and, in particular, large language models, have the potential to bring significant benefits to brands. Still they need to be integrated and developed with safeguards in place to protect the consumer experience and the brand’s reputation. A thoughtful, controlled approach to creating and integrating these powerful models will ensure they perform correctly and deliver amazing consumer experiences.